"B" Admin
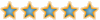

Posts : 1201 Reputation : 4018 Join date : 2010-01-17 Location : undetermined
 | Subject: Latest abrok Development Version Febuary 10th Update architecture to "SFNNv4". Update network to nn-6877cd24400e.nnue. (SF The gift that keeps on giving Thu Feb 10, 2022 7:46 pm | |
| !! latest version !!
Windows x64 for Haswell CPUs Windows x64 for modern computers + AVX2 Windows x64 for modern computers Windows x64 + SSSE3 Windows x64 Linux x64 for Haswell CPUs Linux x64 for modern computers + AVX2 Linux x64 for modern computers Linux x64 + SSSE3 Linux x64 | Author: Tomasz Sobczyk Date: Thu Feb 10 19:54:31 2022 +0100 Timestamp: 1644519271 Update architecture to "SFNNv4". Update network to nn-6877cd24400e.nnue. Architecture: The diagram of the "SFNNv4" architecture: https://user-images.githubusercontent.com/8037982/153455685-cbe3a038-e158-4481-844d-9d5fccf5c33a.png The most important architectural changes are the following: * 1024x2 [activated] neurons are pairwise, elementwise multiplied (not quite pairwise due to implementation details, see diagram), which introduces a non-linearity that exhibits similar benefits to previously tested sigmoid activation (quantmoid4), while being slightly faster. * The following layer has therefore 2x less inputs, which we compensate by having 2 more outputs. It is possible that reducing the number of outputs might be beneficial (as we had it as low as 8 before). The layer is now 1024->16. * The 16 outputs are split into 15 and 1. The 1-wide output is added to the network output (after some necessary scaling due to quantization differences). The 15-wide is activated and follows the usual path through a set of linear layers. The additional 1-wide output is at least neutral, but has shown a slightly positive trend in training compared to networks without it (all 16 outputs through the usual path), and allows possibly an additional stage of lazy evaluation to be introduced in the future. Additionally, the inference code was rewritten and no longer uses a recursive implementation. This was necessitated by the splitting of the 16-wide intermediate result into two, which was impossible to do with the old implementation with ugly hacks. This is hopefully overall for the better. First session: The first session was training a network from scratch (random initialization). The exact trainer used was slightly different (older) from the one used in the second session, but it should not have a measurable effect. The purpose of this session is to establish a strong network base for the second session. Small deviations in strength do not harm the learnability in the second session. The training was done using the following command: python3 train.py \ /home/sopel/nnue/nnue-pytorch-training/data/nodes5000pv2_UHO.binpack \ /home/sopel/nnue/nnue-pytorch-training/data/nodes5000pv2_UHO.binpack \ --gpus "$3," \ --threads 4 \ --num-workers 4 \ --batch-size 16384 \ --progress_bar_refresh_rate 20 \ --random-fen-skipping 3 \ --features=HalfKAv2_hm^ \ --lambda=1.0 \ --gamma=0.992 \ --lr=8.75e-4 \ --max_epochs=400 \ --default_root_dir ../nnue-pytorch-training/experiment_$1/run_$2 Every 20th net was saved and its playing strength measured against some baseline at 25k nodes per move with pure NNUE evaluation (modified binary). The exact setup is not important as long as it's consistent. The purpose is to sift good candidates from bad ones. The dataset can be found https://drive.google.com/file/d/1UQdZN_LWQ265spwTBwDKo0t1WjSJKvWY/view Second session: The second training session was done starting from the best network (as determined by strength testing) from the first session. It is important that it's resumed from a .pt model and NOT a .ckpt model. The conversion can be performed directly using serialize.py The LR schedule was modified to use gamma=0.995 instead of gamma=0.992 and LR=4.375e-4 instead of LR=8.75e-4 to flatten the LR curve and allow for longer training. The training was then running for 800 epochs instead of 400 (though it's possibly mostly noise after around epoch 600). The training was done using the following command: The training was done using the following command: python3 train.py \ /data/sopel/nnue/nnue-pytorch-training/data/T60T70wIsRightFarseerT60T74T75T76.binpack \ /data/sopel/nnue/nnue-pytorch-training/data/T60T70wIsRightFarseerT60T74T75T76.binpack \ --gpus "$3," \ --threads 4 \ --num-workers 4 \ --batch-size 16384 \ --progress_bar_refresh_rate 20 \ --random-fen-skipping 3 \ --features=HalfKAv2_hm^ \ --lambda=1.0 \ --gamma=0.995 \ --lr=4.375e-4 \ --max_epochs=800 \ --resume-from-model /data/sopel/nnue/nnue-pytorch-training/data/exp295/nn-epoch399.pt \ --default_root_dir ../nnue-pytorch-training/experiment_$1/run_$run_id In particular note that we now use lambda=1.0 instead of lambda=0.8 (previous nets), because tests show that WDL-skipping introduced by vondele performs better with lambda=1.0. Nets were being saved every 20th epoch. In total 16 runs were made with these settings and the best nets chosen according to playing strength at 25k nodes per move with pure NNUE evaluation - these are the 4 nets that have been put on fishtest. The dataset can be found either at ftp://ftp.chessdb.cn/pub/sopel/data_sf/T60T70wIsRightFarseerT60T74T75T76.binpack in its entirety (download might be painfully slow because hosted in China) or can be assembled in the following way: Get the https://github.com/official-stockfish/Stockfish/blob/5640ad48ae5881223b868362c1cbeb042947f7b4/script/interleave_binpacks.py script. Download T60T70wIsRightFarseer.binpack https://drive.google.com/file/d/1_sQoWBl31WAxNXma2v45004CIVltytP8/view Download farseerT74.binpack http://trainingdata.farseer.org/T74-May13-End.7z Download farseerT75.binpack http://trainingdata.farseer.org/T75-June3rd-End.7z Download farseerT76.binpack http://trainingdata.farseer.org/T76-Nov10th-End.7z Run python3 interleave_binpacks.py T60T70wIsRightFarseer.binpack farseerT74.binpack farseerT75.binpack farseerT76.binpack T60T70wIsRightFarseerT60T74T75T76.binpack Tests: STC: https://tests.stockfishchess.org/tests/view/6203fb85d71106ed12a407b7 LLR: 2.94 (-2.94,2.94) <0.00,2.50> Total: 16952 W: 4775 L: 4521 D: 7656 Elo +5.21 Ptnml(0-2): 133, 1818, 4318, 2076, 131 LTC: https://tests.stockfishchess.org/tests/view/62041e68d71106ed12a40e85 LLR: 2.94 (-2.94,2.94) <0.50,3.00> Total: 14944 W: 4138 L: 3907 D: 6899 Elo +5.37 Ptnml(0-2): 21, 1499, 4202, 1728, 22 closes https://github.com/official-stockfish/Stockfish/pull/3927 Bench: 4919707 see source |
_________________ looking for Bettina
| |
|